“Mechanisms of artificial intelligence provide real-time fast decision-making based on the analysis of huge amounts of information, which gives tremendous advantages in quality and effectiveness … If someone can provide a monopoly in the field of artificial intelligence, then the consequences are clear to all of us: he will rule the world.” – Vladimir Putin
AI is the Snitch in the geopolitical game of Quidditch. Control the Snitch, control the game. As Putin notes, AI offers endless potential; both good and bad.
Look no further than China, who committed $150B in its effort to lead the world in AI technology.
Abishur Prakash of Scientific American put it bluntly (emphasis mine):
“As nations compete around AI, they are part of the biggest battle for global power since World War II. Except, this battle is not about land or resources. It is about data, defense and economy. And, ultimately, how these variables give a nation more control over the world.”
Semiconductors are the basic building block to any artificial intelligence application. These small pieces of hardware enable AI algorithms to store, run, and test more data.
The rise of AI has flipped the semiconductor industry from a cyclical sector into a secular growth powerhouse. A few statistics point to this secular growth story:
-
- AI-based semiconductors will grow 18%/year over the next 3+ years (5x greater than non-AI chips)
- AI-specific memory chips command 300% higher prices than standard memory chips
- By 2025, AI-related semiconductor technology will account for 20% total revenue
Semiconductor companies will capitalize on these trends by capturing more of the AI technology stack. Under previous technological shifts (smartphones, cloud computing, etc.) semiconductors took smaller portions of the revenue pie (10-20% in most cases).
Here’s the main thing that matters: McKinsey estimates that in an AI-led growth cycle, semiconductors can capture 40-50% of the revenue pie. Remember, AI-based technology will represent roughly 20% of all revenue by 2025. This percentage should increase over time. That means semiconductors will generate 40-50% of a much larger TAM.
This essay will cover three topics:
-
- The AI Technology Stack & Semiconductor Positioning
- How AI Is Changing Semiconductor Hardware Demand & A Few Examples
- Why Investors Should Care
Let’s dive in.
The AI Technology Stack: Semiconductors Will Capture More Share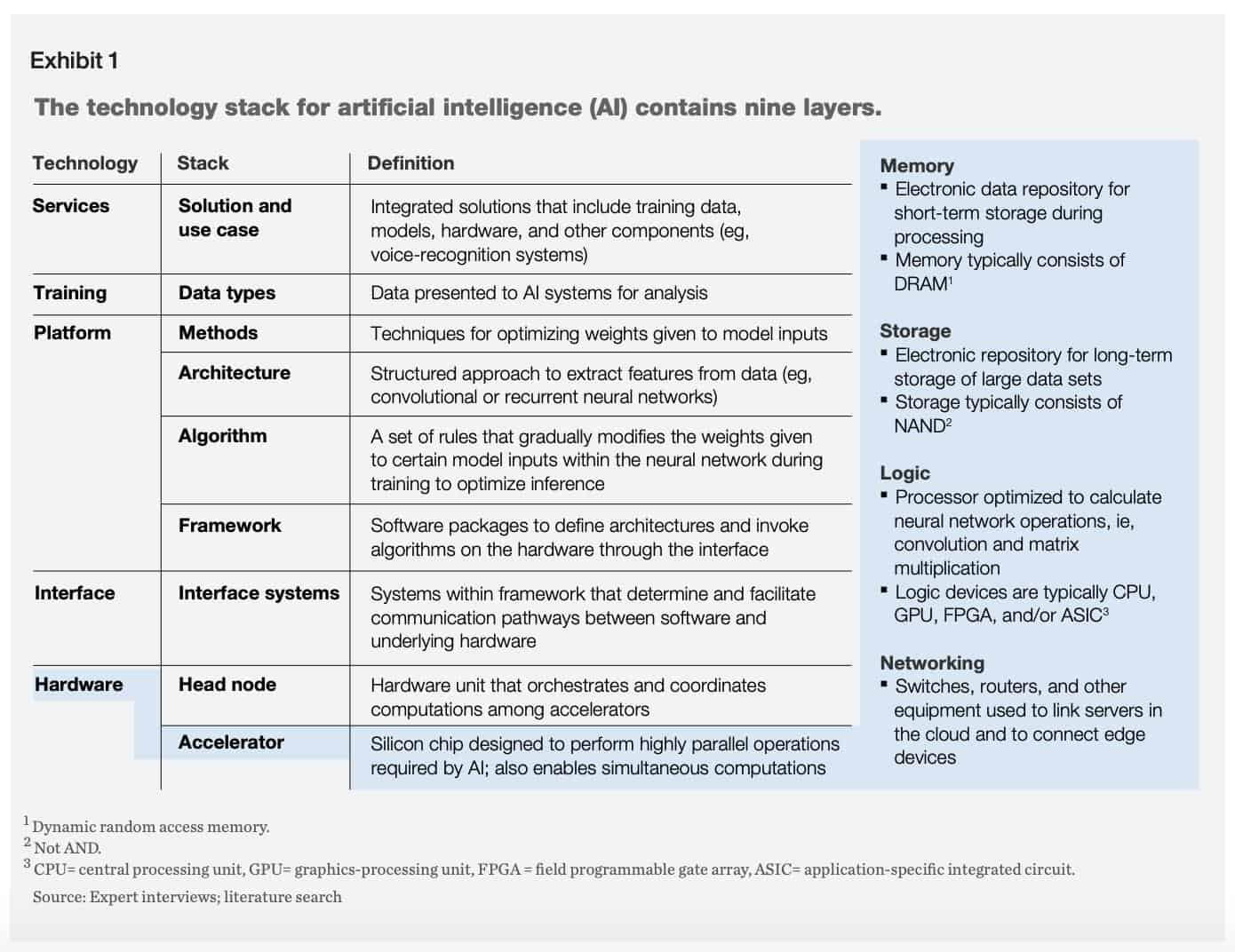
There’s nine layers in the AI Technology stack (see on right). Semiconductors live at the bottom in the hardware layer. Traditionally, this layer meant commoditized products, lower margins and less profitability.
Anyways, these nine layers fixate on two goals:
-
- Train data
- Make inferences from that data
Semiconductors focus on two parts of the stack: head nodes and accelerators. These are the neurons and synapses of the neural network brain.
Your Roadblock is Our Opportunity
According to McKinsey, head nodes and accelerators are the top bottleneck issue for AI developers that want to improve their programs (emphasis mine):
“When developers are trying to improve training and inference, they often encounter roadblocks related to the hardware layer, which includes storage, memory, logic, and networking.”
You can’t run AI programs without these two components. It’s like a human brain. No amount of data matters if you don’t have enough synapses (nodes) to relay information to the brain (accelerator).
It’s this exact reason why semiconductors will switch from a commodity product to a differentiated piece of hardware. Companies will pay more for specialized, AI-specific hardware that makes their programs run more efficiently with less data and computing power.
Why? Because that’s where the roadblock is. It’s not at the software level or any other point in the AI-technology stack. The developer with the best hardware wins.
To understand the dynamics of hardware demand, let’s look at the actual components involved in these AI programs.
The Four Types of AI Chips
AI developers generally have four types of chips at their disposal:
-
- Application Specific Integrated Circuits (ASIC)
- Central Processing Units (CPU)
- Graphics Processing Units (GPU)
- Field-Programmable Gate Array (FPGA)
AI algorithms are increasing the demand for ASIC chips compared to all others. That’s the one we want to pay attention to. Why is this? Allerin offers a few reasons (emphasis mine)
“An ASIC chip is a chip designed to carry out specific computer operations. Hence, ASIC chips are now being developed to specifically test and train AI algorithms. ASICs can be used to run a specific and narrow AI algorithm function. The chips can handle the workload in parallelism. Hence, AI algorithms can be accelerated faster on an ASIC chip. The only major tech giant investing in ASIC chips is Google. Google announced its second-generation ASIC chips called the Tensor Processing Unit (TPU), based on TensorFlow. The TPUs are designed and optimized only for artificial intelligence, machine learning, and deep learning tasks.”
The increased demand will allow semiconductor companies to venture up the technology stack as they build customizable ASIC chips for their OEM partners. We’ll cover that in more detail in the next section.
Now that we know what the AI technology stack is and where semiconductors fall in that stack, it’s time to examine how AI is reshaping semiconductor hardware demand for that all-important last layer.
How AI Is Reshaping Semiconductor Hardware Demand
Gavin Baker’s content was at the top of my reading list when I started my semiconductor/AI research. Baker’s interview with the Market NZZ offers clues at the future of semiconductors, the importance of AI and demand shocks.
Gavin mentioned three separate demand shocks in the semiconductor market:
-
- Virtualization helped utilize servers more efficiently
- Cloud Computing further increased server utilization
- Smartphones cannibalized PC market growth
Now, thanks to artificial intelligence, semiconductors are finally experiencing a positive demand shock. Here’s Gavin’s take (emphasis mine):
“Now, we have a positive demand shock in the form of artificial intelligence … because data quantity is so important for quality, is much more semiconductor intensive.”
He later expands on this thought, saying (emphasis mine), “AI as a demand driver is just getting going. When humans write software, they understand that we want to limit the amount of computational resources. They write software in very elegant ways to minimize compute and memory. Artificial intelligence is none of that. AI is all about semiconductor brute force.”
Semiconductor brute force. AI doesn’t care about computational resources. It cares about maximizing and optimizing its algorithm to make better decisions. What’s needed in that environment? More storage, more capacity and higher memory bandwidths.
McKinsey’s semiconductor/AI research reaffirms Gavin’s thoughts. In their article, Artificial-intelligence hardware: New opportunities for semiconductor companies, McKinsey notes how much data storage AI applications will require (emphasis mine):
“AI applications generate vast volumes of data—about 80 exabytes per year, which is expected to increase to 845 exabytes by 2025. In addition, developers are now using more data in AI and DL training, which also increases storage requirements. These shifts could lead to annual growth of 25 to 30 percent from 2017 to 2025 for storage—the highest rate of all segments we examined.”
Deep learning and neural networks are driving most of the data demand. McKinsey explains, saying (emphasis mine), “AI applications have high memory-bandwidth requirements since computing layers within deep neural networks must pass input data to thousands of cores as quickly as possible.”
The changes in demand drivers mainly affect four categories within the semiconductor space: compute, memory, storage and networking. Let’s see what the future will look like for these categories in an AI-first world (data from McKinsey).
Compute: Deep Learning Takes Share in Data Centers & Edge
There’s two areas we should consider when analyzing the computing space:
-
- Data centers
- The ‘Edge’
Both spaces have seen tremendous growth in training and inference hardware. This makes sense as all ML/DL need to train and test their algorithms. Data centers will see double the demand for training and 4-5x the demand for inference hardware.
The edge poses even greater demand needs. In 2017, Edge inference and training markets combined for a total of <$0.3B. By 2025, those markets will grow to a combined $5-$6B! That’s the power of AI-driven demand shocks.
ASICs chips look ready to fulfill this increased demand. McKinsey estimates that by 2025, ASICs chips will command 50% of data-center usage and 70% of edge application usage.
Memory: High-Bandwidth & On-Chip Will Dominate
AI is shifting memory demands away from DRAM (dynamic random access memory) to two other sources:
-
- High-bandwidth memory (HBM)
- On-chip memory (OCM)
HBM let’s AI algorithms process more data, faster. This no-brainer solution led two of the largest technology companies (NVDA and GOOGL) to switch their preference to HBM vs. DRAM. That switch comes at a cost as HBM is 3x more expensive than DRAM. But again, in an AI-based world, these chips become differentiators — not commodities.
AI is also changing the way memory is stored at the edge. More companies are switching to on-chip memory devices as a way to save both space and money. Consider GOOGL’s TPU. Developing that one ASIC saved the company billions of dollars in opening up new data center storage units.
Now that it’s easier to store memory for edge devices to generate inferences, it’s sparked a rise in edge-based inference projects. Paul McClellan, writer for SemiEngineering.com elaborates on this phenomenon (emphasis mine):
“As AI algorithms moved out into edge devices such as smartphones and smart speakers, there was a desire to do more of the inference at the edge. The power and delay inherent in uploading all the raw data to the cloud was a problem, as are privacy issues. But inference at the edge requires orders of magnitude less power than a data center server or big GPU. In turn, that has led to a proliferation of edge inference chip projects.”
Looking out 5-10 years, on-chip should command a much higher percentage of the total memory market as more companies move to edge computing/inference.
Storage: Training Will Dominate Storage Needs
When you’re training an algorithm you need all the data you can get. Yet at the inference level, the data requirements drop off significantly.
This fluidity in storage needs creates an opportunity for new storage technologies. Specifically, new forms of non-volatile memory (NVM). Think of NVM as long-term memory. It’s the anniversaries, birthdays, and ticker of your worst-performing stock.
McKinsey sees storage demand increasing 25-30%/year for the next five years as AI-based programs consume more data at the edge and in data centers.
Networking: Speed Kills — Avoid Bottlenecking
Networking is extremely important in an AI-first world. Networks connect multiple servers to each other to perform the important task of training a model. Slow network speeds reduce the AI’s ability to train its model.
This can have devastating effects in autonomous driving, where edge programs need to make decisions in real-time. Staying with autonomous driving, McKinsey estimates it takes roughly 140 servers to reach 97% accuracy in obstacle detection. Network speed could mean the difference between a vehicle slamming a guard rail or cruising home unscathed.
McKinsey sees two viable options for potential AI networking issues:
-
- Programmable switches to route data in different directions (like the Mellanox Quantum™ CS8500 HDR Director Switch Series)
- Using higher-speed interconnections in data servers
AI In Action: What We’re Excited About
We now know a few things about AI. We know what the technology stack looks like. We know where semiconductors fit in that stack and how AI is reshaping the hardware demand cycle. We also know the four main areas of semiconductor hardware that will see disruption and innovation from AI-based technologies.
Let’s cover a few real-life areas where we’re excited about the future of AI application.
The below use cases were taken from this devprojournal article.
Application 1: Education
AI has many applications in the underearning education industry. AI can tackle most of the monotonous tasks a teacher performs. This leaves the teacher free to do what he does best, teach kids.
There are also opportunities for AI-enabled tutoring. Companies like Content Technologies use AI to turn large, complicated textbooks into easy-to-read flashcards, note packets and practice exams.
COVID-19 has also accelerated the role online learning plays in a person’s education. Carnegie University developed its own software to provide remedial classes to incoming freshman and sophomores — saving billions of dollars in tuition fees.
Application 2: Healthcare
Healthcare is arguably the most popular space for AI-based interventions. There’s a few that excite us, including:
-
- Tumor/disease diagnosis
- Symptom checkers
- Clinical study pop-ups based on radiology image results
- Medication trackers
You can find 28 more examples of AI in healthcare here.
Application 3: Finance
The finance companies that win over the next 5-10 years will employ AI-first business models. Applications vary from fraud detection, loan processing, credit risk assessments and advertising.
Cardlytics (CDLX), a Macro Ops portfolio holding, does just that. Using AI to sift through millions of card/bank transactions, CDLX is able to provide marketers with advertising channels and consumers with deals from brand partners.
These three applications bring us to our final section: why you should care.
Why Should We Care About Semiconductors?
Semiconductors and AI are in the early stages of an exponential, long-term growth trend. We don’t know which companies will win in the end. But what we do know is that this is one of the best spots to fish over the next decade.
Why? The market is horrible at pricing long-term exponential growth. It’s too short-term oriented to see the wave forming way out in the ocean. Mr. Market’s focused on next quarter and next year. Not on the major shifts happening over the next five years.
Mr. Market’s ignorance is our opportunity. His short time-frame is our alpha. By understanding the drivers of this long-term secular growth trend, we can position ourselves to invest in these areas now.
McKinsey estimates that the AI-spurred semiconductor market will grow ~18%/year for the next five years (see right). Things get better when you look at specific areas like storage, which will grow near 30%/year for the next five years.
The bull thesis is simple:
-
- AI-based technologies will represent larger and larger portions of total semiconductor revenue
- Semiconductors should get 40-50% of this growing AI-specific revenue base (as opposed to 10-20% in other cycles)
- The shift from cyclical to secular growth will result in smoother revenues and earnings, which will lead to higher multiples.
Next week we’ll explore these high-growth semiconductor spaces in greater detail. We’ll reveal why industries like storage are set to pop and what companies we should invest in to capture those trends.
We’re excited about this space and the long-term growth prospects. Moreover, some of the long-term charts for these semiconductor companies are setting up for much higher prices. And we’ve got our eyes on under-the-radar companies taking advantage of these new technologies with interesting business models.
See you next week!